An exceptional discount of 10% is applied on all registration fees this year.
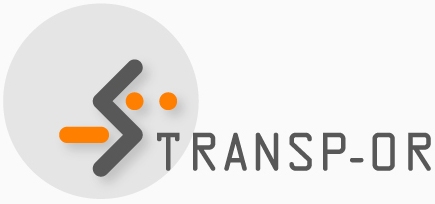
The course is organized by the Transport and Mobility Laboratory, EPFL.
Deadlines
Scholarship applications: | November 22, 2024 |
Early registration: | January 17, 2025 |
Late registration: | January 24, 2025 |
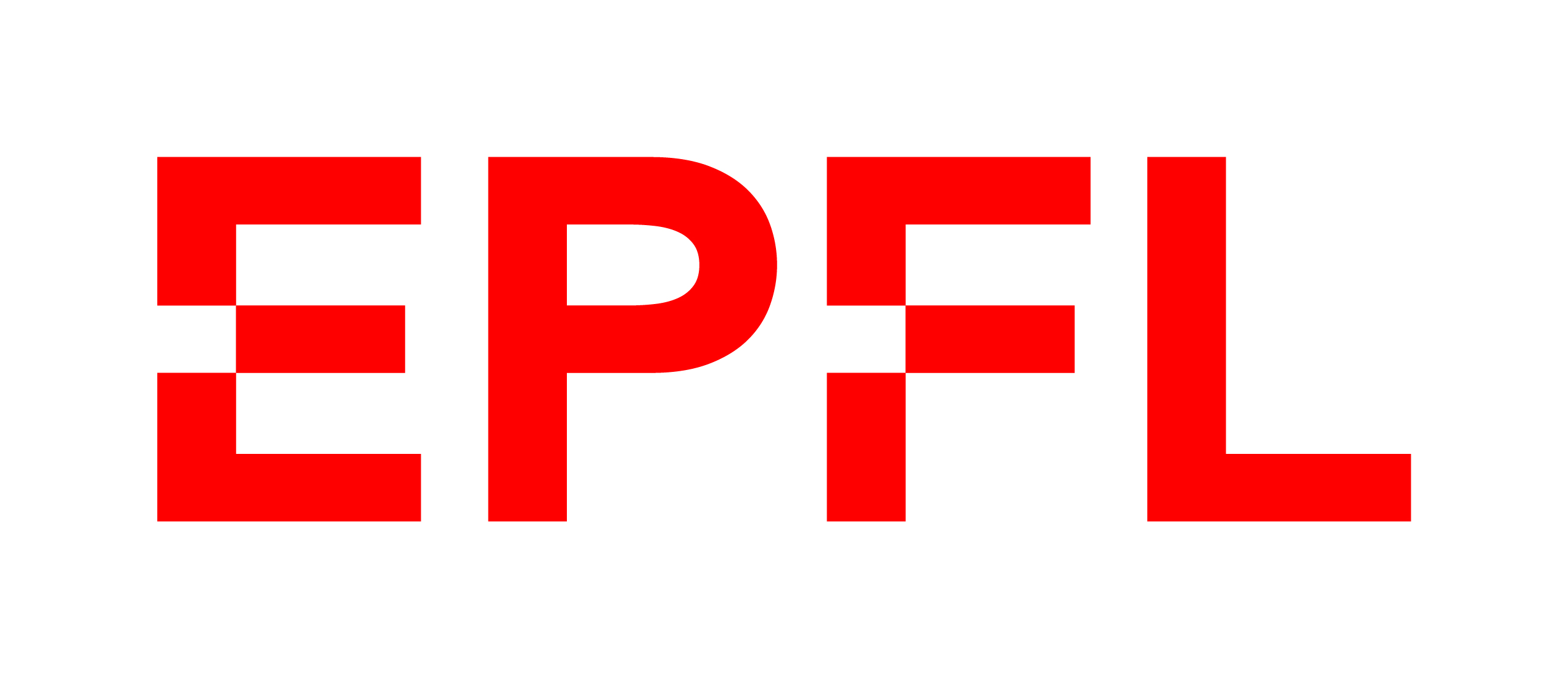
Discrete Choice Analysis: Predicting Individual Behavior and Market Demand
Accurate predictions of the demand and market shares are critical for a wide variety of businesses and public organizations. Examples of applications include: predicting demand for a new product under alternative pricing strategies; designing a business plan for a new technology; analyzing the impact of a merger on market shares; forecasting the ridership on a new metropolitan transit service; and analyzing competitive scenarios for introducing a new telecommunication service. To accomplish these tasks, discrete choice analysis provides powerful methodological tools. Based on the modeling of individual behavior, it is used to model in detail the structure of a market, and to predict the impact of various scenarios.
This one-week program undertakes an in-depth study of discrete choice models and their applications. It provides participants with the practical tools necessary for applying new discrete choice techniques. By examining actual case studies of discrete choice methods students will be familiarized with problems of data collection, model formulation, testing, and forecasting and will gain hands-on application experience by using readily available software to estimate and test discrete choice models from real databases. The course will emphasize applications of discrete choice methods to strategic and tactical marketing and to policy-related problems.
The course has been designed by Prof. Ben-Akiva who is offering it every summer at the Massachusetts Institute of Technology. It is organized in Europe by Prof. Michel Bierlaire, from the Transportation and Mobility Laboratory at EPFL.
Who should attend?
The course is designed for professionals (from industry and public authorities) and academic researchers (professors, researchers, PhD students), interested in understanding and predicting consumer choices, demand and market share, such as marketing analysts, managers, planners, economists, engineers, operations researchers.
Topics covered
The course will cover the following topics:
- Fundamental methodology, e.g. the foundations of individual choice modeling, random utility models, discrete choice models (binary, multinomial, nested, cross-nested logit models, MEV models, probit models, and hybrid choice models such as logit kernel and mixed logit);
- Data collection issues, e.g. choice-based samples, enriched samples, stated preferences surveys, conjoint analysis, panel data;
- Model design issues, e.g. specification of utility functions, generic and alternative specific variables, joint discrete/continuous models, dynamic choice models;
- Model estimation issues, e.g. statistical estimation, testing procedures, software packages, estimation with individual and grouped data, Bayesian estimation;
- Forecasting techniques, e.g. aggregate predictions, sample enumeration, micro-simulation, elasticities, pivot-point predictions and transferability of parameters;
- Examples and case studies, including marketing (e.g., brand choice), housing (e.g., residential location), telecommunications (e.g., choice of residential telephone service), energy (e.g., appliance type), transportation (e.g., mode of travel).
Prerequisites
It is assumed that participants have a basic knowledge of statistical methods, including linear regression models. No a priori knowledge of discrete choice models is needed. Basic topics are covered early in the week, while more advanced topics are covered later. An introduction to the software package BIOGEME that will be distributed at the course will be provided during the first lab, prior to working on the case studies. It may be useful to review basic statistical methods in a textbook such as
R. J. Larsen and M. L. Marx (2010) An Introduction to Mathematical Statistics and Its Applications (5th Edition), Prentice Hall (chapters 1 to 6).
An introduction to Discrete Choice Models is available as an online course on the edX platform. It is advised to review the online material before coming to the course. Although not required, it may help the participants to go deeper into the material, and increase the benefits of the participation to the course.
Schedule
Time Slot | Sunday, January 26, 2025 | Monday, January 27, 2025 | Tuesday, January 28, 2025 | Wednesday, January 29, 2025 | Thursday, January 30, 2025 |
---|---|---|---|---|---|
09:30 - 11:00 | Introduction, Choice Behavior and Discrete Choice Models Ben-Akiva | Nested Logit Models Bierlaire | Mixture Models Bierlaire | Discrete Panel Data Bierlaire | Bayesian Estimation Ben-Akiva |
11:15 - 12:45 | Specification and Estimation of Logit Models Ben-Akiva | Extreme Value Models; Aggregate Forecasting and Microsimulation Bierlaire | Simulation-Based Estimation Bierlaire | Machine Learning with Theoretical Constraints Ben-Akiva | Online Personalization and Optimization Ben-Akiva |
14:15 - 15:45 | Specification Testing, Machine Learning and Regularization Ben-Akiva | Endogeneity; Sampling and Estimation Ben-Akiva | Stated Preferences Methods Ben-Akiva | Mixture Models with Latent Variables Ben-Akiva | Questions & Answers Ben-Akiva/Bierlaire |
16:00 - 17:30 | Computer Lab I: Introduction; Logit Estimation and Testing | Computer Lab II: Nested Logit; Aggregate Forecasting | Computer Lab III: Logit Mixtures; Combining Data (SP and RP) | Computer Lab IV: Hybrid Choice Models | Computer Lab V: Hierarchical Bayesian Estimation; Individual Prediction |
Welcome reception: | Sunday, 17:30 |
Dinner: | Wednesday, 19:00 |